The real problem with AI isn’t sentience, it’s privacy
Ketch’s chief innovation officer Yacov Salomon writes that responsible AI will require us to rethink data privacy. Here’s what you need to know.
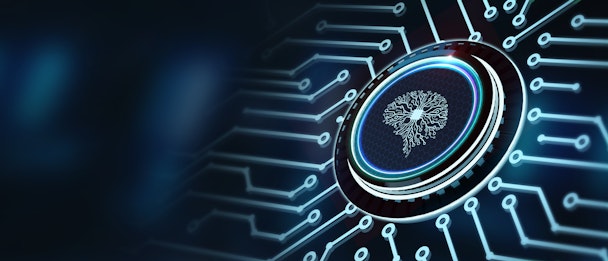
Artificial intelligence is built from data / Adobe Stock
Surprise, surprise, Google has fired the engineer who claimed his LaMDA chatbot had achieved consciousness and feared being turned off. It’s easy to see why Google found the episode embarrassing: the media jumped on the story, sparking widespread discussions about the ethics of exploiting ’sentient’ algorithms.
The reality is that we’re still a long way from any kind of machine sentience. Today’s algorithms are good at certain narrowly defined tasks, but they lack general intelligence or anything approximating self-awareness.
Still, there’s another area where AI really does present an ethical problem: data privacy. That’s a much bigger deal than sentient machines because, without real trust in the way our data is used and safeguarded, consumer skepticism could wind up slamming the brakes on the AI revolution.
Why data privacy?
Many people are perfectly happy sharing their data via AI interfaces. I’m quite happy to tell my healthcare provider’s diagnostic chatbot that I have a headache, for instance, or to give my address and payment info to an e-commerce tool. Protecting such data isn’t trivial, but it’s manageable using existing data privacy regulations and technologies.
The bigger issue comes when you look under the hood. Modern AI algorithms get their power from the vast volumes of data upon which they’re trained. It’s now possible to sculpt algorithms capable of identifying a cat – or a suspected criminal – by feeding them mountains of data scraped from public and private sources.
Why is that a privacy concern? There are two key reasons.
First, the datasets themselves are often gathered with scant regard for the rights of the data subjects. That’s partly because such datasets frequently contain things we don’t usually think of as data – photographs, for instance, or the biometrics of the people shown in those photos. Creating new regulations and standards to govern the use of widely circulating data – including images, behavioral data and more – will be crucial as AI tools become increasingly mainstream.
Secondly, building AI doesn’t just require using more data and more kinds of – it also requires systems of processing and embedding data that regulators have never previously contemplated and that our existing data privacy systems were simply never intended to manage.
AI is built from data
Every modern AI tool is literally built from data. Algorithms are the direct product of the datasets used to sculpt and train them – and that means data persists in the AI systems that it was used to build. In many cases, moreover, the AI training process can now be reverse-engineered: by reading the traces left in an algorithm, it’s possible to recover or reconstruct the data that was originally used to train a given AI model.
This isn’t just a theoretical concern. Model inversion attacks are already being used to extract recognizable facial images from algorithms trained using biometric data. Natural language AI tools are also vulnerable: Google engineers found a predecessor of their ’sentient’ LaMDA tool could be manipulated to extract personally identifiable information including email addresses, phone numbers, chat messages and more.
Training data isn’t used once and then safely disposed of. It lingers on, embedded in and potentially extractable from the finished AI product – and as algorithms are trained on bigger and bigger datasets, the potential for data leakage becomes greater and greater. The risk is real and we need to act now to secure our data in an era of ubiquitous AI.
Time for a new approach
First, we’ll need to recognize that we currently have a serious blind spot when it comes to ethical AI and data privacy. Every aspect of the AI sausage factory – from data collection, to training, to deployment – needs careful scrutiny from both regulators and tech creators to ensure that data is properly protected at every step of the way.
In part, that will depend on front-end privacy protections such as the use of new cryptographic methods – including federated learning, differential privacy and homomorphic encryption – to protect data and implement robust responsible AI within classical AI frameworks. Such methods, already used in tools such as Siri and Alexa, could allow AI systems to be trained without giving them unfettered access to data, providing a measure of protection for data subjects.
Even with such innovations, though, AI developers will need to keep their privacy responsibilities front of mind. What happens, for instance, if a data subject revokes consent? It’s no longer enough to simply delete their data – we’ll also need to ’untrain’ AI tools so that the user’s data can’t be extracted from the underlying algorithm. That’s a solvable problem, but only if we insist upon such solutions being developed and implemented.
Finally, we’ll need to clearly articulate to users exactly how their data is being used and how it’s being imprinted in AI systems. Today’s consumers are increasingly willing to grant access to their data in exchange for new functionality, but they expect transparency and agency at every step of that process. This is a balancing act and it will take time to get it right. But consumers are paying attention and AI developers that ignore data privacy will be punished both by regulators and in the marketplace.
Trust is key
There’s really no need to worry (yet) about the ethics of sentient AI algorithms. But we should be paying close attention to the impacts that AI tools are having on our data privacy.
This isn’t just a question of ethics; it’s a crucial step towards developing and scaling transformative new AI technologies. If people don’t trust AI, they won’t willingly share their data with AI developers, and as that lack of trust spreads it could hamstring the sector’s development.
The bottom line is that in the new data economy, trust is the key to winning data access and driving innovation. To realize the potential of current and future AI technologies, we need to give people meaningful end-to-end data privacy protections and that means building technological and regulatory systems to manage and secure the data upon which AI depends.
Yacov Salomon is the co-founder and chief innovation officer at Ketch and a recognized authority in the fields of machine learning and AI. For more, sign up for The Drum’s daily US newsletter here.